Osteo-BCNN
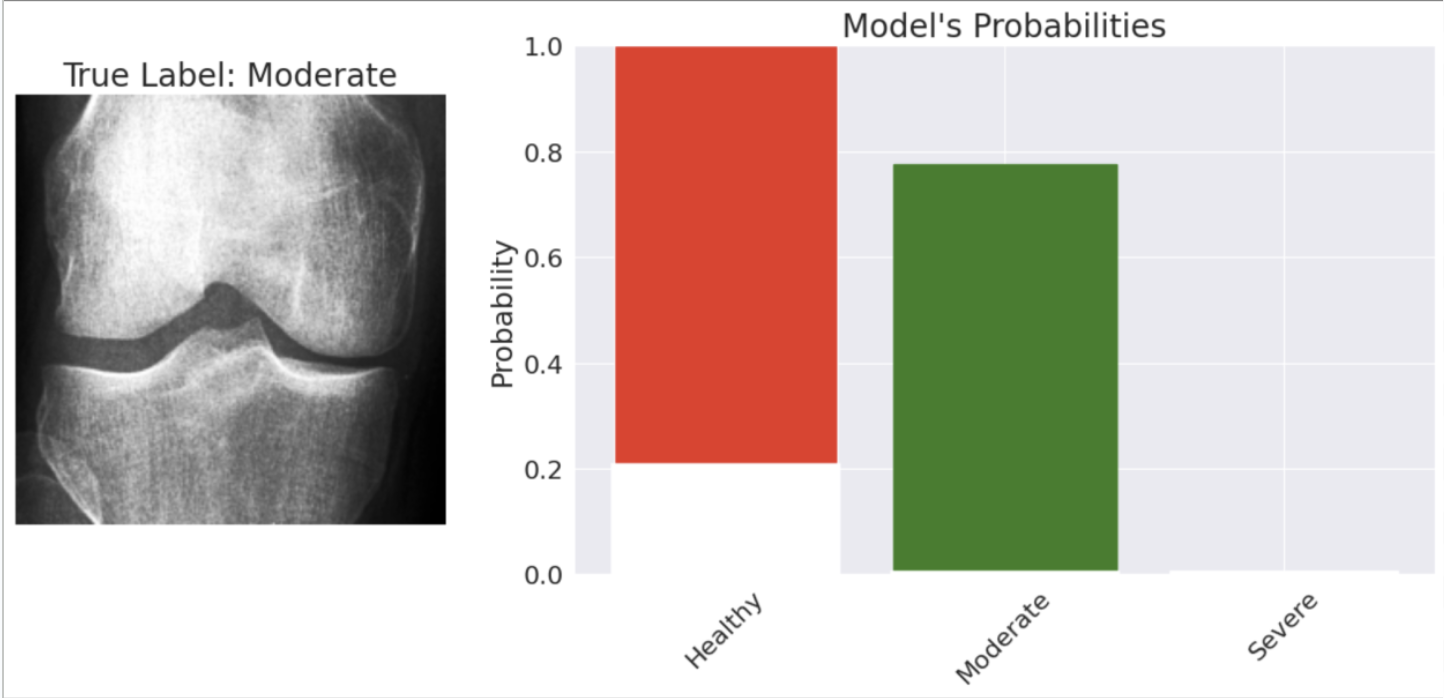
Abstract
Osteoarthritis (OA) is a prevalent joint disease in the knee which is the most common form of arthritis. Accurate diagnosis and severity assessment are crucial for effective treatment. However, existing AI models can yield incorrect predictions, leading to potential harm. Our research addresses this issue by introducing Bayesian Convolutional Neural Networks (BCNNs) to quantify prediction uncertainty, so results with high uncertainty can be disregarded. Our study focuses on OA severity assessment using knee X-ray images. We propose a generalized approach applicable to diverse patient populations. By incorporating Bayesian techniques, we enable uncertainty estimation for each prediction. We trained BCNNs on a large dataset of knee X-rays, capturing both structural features and uncertainty. Our model accounts for epistemic and aleatoric uncertainty, allowing clinicians to gauge the reliability of predictions. To enhance the robustness and generalization of our model, we incorporated data augmentation techniques during training. Our BCNN model achieved 85% accuracy in OA severity prediction. By providing uncertainty estimates, medical professionals can make informed decisions, avoiding undue reliance on potentially flawed AI outputs. This research has significant implications for improving patient care and reducing misdiagnoses.